IMPORTANT UPDATE
Edanz Expert Editing Is Now Scribendi Scientific Editing
As of 30-September-2024, Edanz Expert Editing has transitioned to our sister company, Scribendi, where you'll find our new Scientific Editing service. This move allows us to continue providing the same high-level expertise you've come to expect, now on a more streamlined and improved platform.
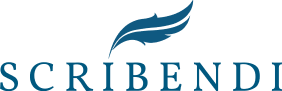
What is Scribendi Scientific Editing?
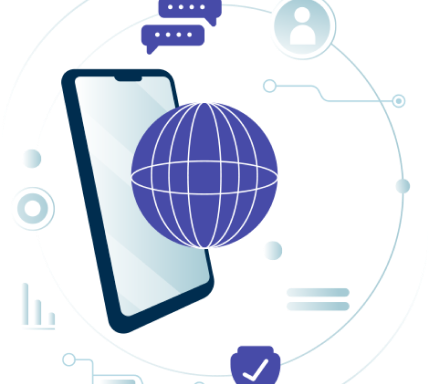
- The same high-level expertise you've come to expect
- The same editors - experienced researchers editing and reviewing your manuscript
- Improved scientific accuracy, clarity, and content
- Tailored recommendations for top journal submissions
- A better price! That's right, you get the same great service for a lower price!
Lower Cost With The Same High Quality Editing
2,500 Words - 48-Hour Turnaround
Edanz
Expert Editing
$545
VS
Scribendi
Scientific Editing
$235
NOTE: Prices shown are examples. Actual pricing may vary based on confirmed word count, delivery time, and other factors.
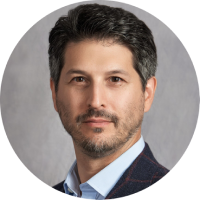
Thomas da Costa
CEO, Edanz and Scribendi
I'm thrilled about our move to Scribendi! This transition lets us offer the same trusted high-quality editing at a more affordable price.
Our dedicated expert team will continue to support your publishing success with a more efficient, user-friendly platform.
We Understand You May Have Some Questions About This Change
Is Edanz going away?
No. Edanz and Scribendi are two brands within the same organization. By making this change,
we’ll be
able
to focus each brand and service to the markets for which they are the best fit.
If the service is lower cost does that mean the quality isn’t the same?
No. The high-quality editing that you enjoy today with Edanz Expert Editing will be the same with
Scribendi Scientific Editing. The service will use the same editors as before. Each paper will
go through the same thorough quality check
As an existing Edanz customer, will I have an account on the Scribendi website?
Yes! We’ve set up an account for you on the Scribendi website. You can login here using your Edanz account
email address.
You will need to create a new password when accessing your Scribendi account for the first time.
Other Questions?
Our dedicated customer support team is here to assist you. If you have any questions, please reach out to them: global@edanz.com